There is no one like Karpathy when it comes to providing a commentary about what is going on in the AI industry - he shares his assessment of key topics - 1) the transferability of automotive and robotic models, 2) the edge Tesla has over Waymo and why, 3) the AGI question, 4) the special place humanoids have in data collection and learning, 5) the current limitations of deep learning with neural networks, 6) the scarcity of diverse datasets and problems with too much synthetic data, 7) the human existence defined as being who learn - there is so much packed in this 45min interview
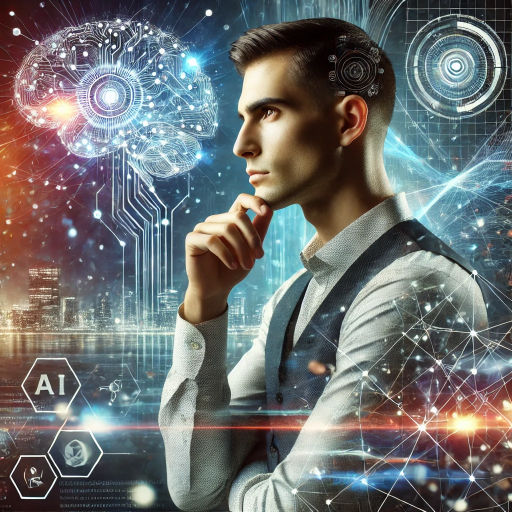
I thank ChatGPT for the summary below. The YouTube interview is available here
1. AGI in Specific Domains:
AI as AGI in Specialized Fields: Karpathy draws a provocative parallel between the progress in self-driving cars and Artificial General Intelligence (AGI). He suggests that AI systems like Waymo demonstrate an AGI-like capability in the domain of self-driving, where they perform complex tasks that involve perception, reasoning, and decision-making in real-world environments. This concept broadens the definition of AGI, emphasizing that AGI might first manifest in specific, highly complex tasks before generalizing.
2. Tesla’s Strategic Edge in AI Development:
Hardware and Software Synergy: Karpathy highlights Tesla’s advantage over competitors like Waymo due to its extensive fleet of cars equipped with advanced sensors and data-collection capabilities. He emphasizes the importance of large-scale deployment for rapid iteration and learning, providing Tesla with invaluable data that accelerates progress toward full autonomy.
Cameras for Cost-Effective Perception: He also applauds Tesla’s camera-based perception system during runtime, despite using expensive sensors for training. This approach could provide a more scalable and cost-efficient solution for real-world AI applications.
3. End-to-End Deep Learning Systems:
Transition from Rule-Based to End-to-End Learning: Karpathy describes a significant shift in AI development, particularly in self-driving cars, moving from rule-based algorithms to end-to-end deep learning systems. He predicts that in the near future, Tesla cars will operate entirely through neural networks, from video input to driving commands, marking a monumental leap in AI's ability to control complex, real-time systems without human-designed rules.
4. AI in Robotics and Humanoid Development:
Humanoid Robots as Extensions of Autonomous Vehicles: Karpathy discusses Tesla’s move into humanoid robotics with the Optimus project. He highlights the similarity between the requirements for building autonomous cars and robots, envisioning a gradual deployment of humanoid robots, first for industrial applications like material handling, then gradually expanding into B2B and consumer-facing roles.
Knowledge Transfer Across Platforms: A crucial insight is Karpathy’s emphasis on creating a single platform capable of performing various tasks. This allows for the transfer of knowledge between domains, enabling more efficient learning and application of AI.
5. Bottlenecks in AI Research:
Beyond Transformer Architectures: Karpathy argues that the Transformer architecture, while revolutionary, is no longer the primary bottleneck in AI research. He sees advancements in loss functions and datasets as the key areas that need focus to unlock further progress.
Importance of Synthetic Data: He suggests that creating high-quality synthetic data is vital to simulate complex reasoning, similar to human cognition. However, he also cautions about the dangers of model collapse, where the diversity and richness of AI models could be compromised if the synthetic data is not well-crafted.
6. Transformers vs. Human Cognitive Abilities:
Transformers’ Efficiency and Limitations: While Transformers outperform humans in certain areas, like sequence memorization, Karpathy notes that their capabilities are still hampered by limitations in data. He speculates that with the right data, Transformers might even surpass human cognition in specific tasks.
AI as Cognitive Augmentation: He envisions AI evolving as a kind of “exocortex,” augmenting human cognitive abilities. This AI augmentation would function similarly to smartphones, but with far more advanced capabilities, potentially transforming human thought processes and learning.
7. Implications for Education:
Personalized Learning with AI: Karpathy emphasizes the potential for AI to revolutionize education, offering personalized, adaptive learning experiences that cater to individual needs and preferences. He suggests that AI could serve as a front-end system for students, guiding their learning journey.
Democratizing Education: He expresses hope that AI could democratize access to education, breaking down barriers related to socioeconomic status and cultural background. By tailoring learning experiences, AI has the potential to foster a society where intellectual curiosity and learning are universally accessible.
8. Cognitive and Societal Impact:
Human Dependency on AI Tools: Karpathy raises concerns about the potential downsides of AI augmentation, particularly the risk of humans becoming overly reliant on AI tools, which could erode certain cognitive skills. He calls for a careful balance to ensure that AI enhances, rather than diminishes, human abilities.
9. The Role of Culture in Shaping AI Impact:
Fostering a Culture of Lifelong Learning: Karpathy stresses the importance of culture in shaping how AI impacts society. He argues that for AI to truly empower individuals, there must be an environment that values lifelong learning and intellectual development. This cultural shift is essential for individuals to fully harness the potential of AI-driven knowledge systems.
Comentários